Racism, Redcards, and Rabbit Holes
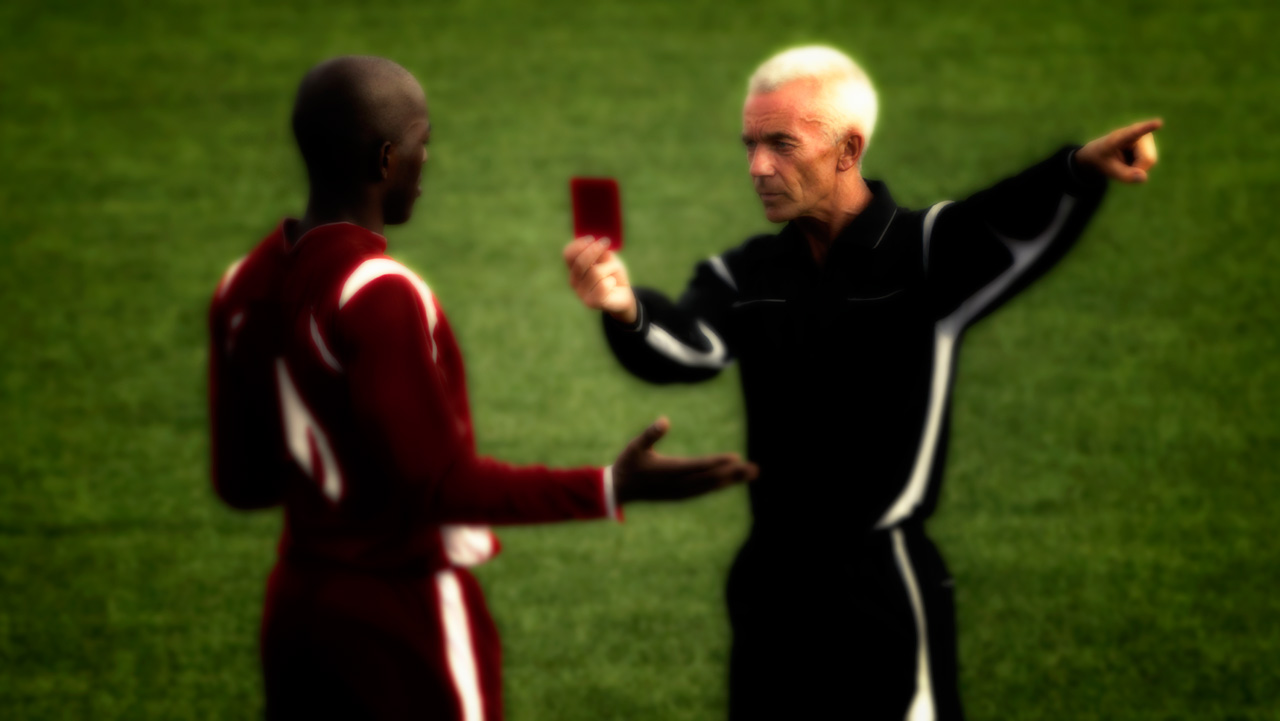
Statisticians have warned for a long time that inexpert modeling can produce distorted statistical results. The replication crisis in science has often been attributed to poor statistical practice with part of the cure being to employ more statisticians. But would this really help? An interesting study by Silberzahn et. al. was posted earlier this year that looked at how consistent statisticians themselves were in their analysis.
The team of researchers obtained a data set, formulated a question, and then posed a statistical challenge by asking different teams of statisticians to produce an analysis. They wanted to see if the teams would agree on both their approach and their results. The answer appeared to be “Not by a long way!”
An interesting question
The question they posed was interesting in itself (and likely to court controversy): “Are darker skinned football (soccer) players more likely to be given red cards than lighter skinned players?”
For this, they assembled a data set of players in European football leagues. Because an individual referee might be particularly prone to bias, they also wanted to look at referees, although these were anonymized. Their final data set consisted of pairs of players and referees, the number of times they had been in the same game, and the number of red cards the player had received from the referee. They also included a number of other possible explanatory factors: player position, age, height, weight, and statistics about their performance, current club, and league. Along with this, they included the referee’s country of origin—the sort of things you might think could also be important in getting red cards.
The authors convinced 29 teams of statisticians to try to use these data to answer this question. They even had a fairly extensive process of consultation and discussion after an initial analysis had been done. Nonetheless, the analyses that different teams chose were very different and so were their resulting conclusions.
First, there was a wide range of conclusions, from “Having darker skin doubles your chance of getting a red card” to “Having darker skin slightly decreases your chance of getting a red card.” That said, the bulk of the teams found that darker skinned players had about a 30 percent increased chance of a red card. While this seems large, the fact that red cards are very rare means that the absolute increase in the rate of red cards was pretty small.
Some teams found statistically significant results in terms of the increased risk of a red card, whereas among the teams that estimated a lower risk for darker skinned players, none found that the difference was statistically significant. The authors of the statistical challenge noted that if you just chose one of the participating statistician teams by chance (and most studies only use one statistician), you’d have a 61 percent chance of producing a statistically significant result.
Part of what drove these different conclusions was the diversity of models employed. While the problem being investigated appears simple, there are many ways it can be analyzed. For example, linear regression just tries to predict the number of red cards based on skin color and possibly other factors, whereas Poisson regression treats the number of red cards as a count (i.e., an integer) but imposes additional assumptions about how the number of cards changes with skin color and other covariates. Logistic regression uses a model in which we have a number of red cards out of the number of games played, and uses different assumptions than Poisson regression. All three basic models were tried by various teams.
Another modeling choice was whether to account for systematic differences between players: A particularly aggressive player might tend to get more red cards, a timid one might get fewer, and since we have more than one record for each player, these counts are correlated. There are a number of ways to account for this. Similarly, referees may also be systematically different from one another.
Even more surprising was how much the teams differed in which other factors (age, height, position, league, etc.) they controlled for. No statistical team used everything, and out of 29 teams there were 19 different combinations of factors employed. Some teams employed no factor in common with others. Most teams did spend some time looking at what factors “should” be important: So these statisticians not only didn’t agree on how much difference skin tone makes, they couldn’t agree on how much difference anything makes!
The lessons that the authors draw from this study are something of a doom-and-gloom kind: if even different statisticians can’t arrive at the same conclusions, is there any hope? Or maybe we should demand independent analysis from several statisticians—a boon to statistical employment!
It certainly is the case that, while statisticians attempt to control for variability (“What answers might you get if you did this again?”), there aren’t good tools for controlling the variability of modeling choices. This is true even when we design algorithms to select models, let alone trying to work out what happens in statisticians heads! This study suggests that differences among statisticians (which we don’t quantify) are possibly as important as the sort of variability they try to analyze.
On the other hand, I think the authors rather overstate how bad things are. First of all, this was a difficult test-case: The data are very noisy and do not measure the causes that we would like to look at; only associations. Almost every team’s report included a passage on quality issues, and the effect is only ever “just” significant in most analyses—flirting with 0.05 on one side or another. This means that if red cards are given out by chance, the likelihood of seeing these data (or more extreme) is sometimes a little less than 5 percent and sometimes a little more than 5 percent resulting in “statistical significance” in the first situation and not reaching statistical significance in the second. But while the conclusions may seem disparate, the close p-values suggest similar conclusions.
Second, we generally want our results to be robust to statistical analysis. Good statistical practice is to try several (realistic) models and only report significance if they all give the same type of result; some careful thought is needed before you exclude any model. In this case, most of the models pointed towards a small bias against darker skinned players. The most extreme results (very large effects, or negative effects) were all associated with unusual modeling strategies.
For example, one team used a technique that removed most of the records with no red cards (which is also most of the data) and just focused on those records with one or more, which meant basically modeling how many red cards were given to players who got at least one. Among the more “standard” models, there was still disagreement about the significance of the trend. This is the natural result of an effect that is only ever just significant (or just not so), along with choices like whether to include “red cards resulting from two yellow cards in one game,” or how to combine two ratings of a players skin color.
One thing that the study did not emphasize very much was a secondary question: It was hypothesized that referees from countries that have higher racial bias might be more prone to giving red cards to darker-skinned players. In contrast to the first question, which just asked whether darker skinned players tended to get more red cards, this asks whether certain referee characteristics are associated with giving more red cards to darker skinned players.
To assess this, the authors obtained scores of implicit bias and explicit bias from the referee’s country of origin using aggregated results from Project Implicit, and they shared these with each statistical team. Almost all teams approached this question of whether these biases made referees more or less prejudiced using the same additional modeling components, and none of them felt that there was any evidence to support the statement. So, on half of the challenge’s questions, there was unanimous agreement.
Model quality control
Despite so much variability in the teams’ conclusions, the challenge authors left out one crucial assessment: model quality. Clearly, some models do a better job of describing data than others, and if you leave out something important you are unlikely to have a good model. Perhaps the better models (i.e. exhibiting more agreement with the data) all pointed to a large effect; or maybe vice versa. Not all the models can be easily compared, but there are some tools to measure model quality and it would be useful to see if some of the more extreme results really do represent “as good” a conclusion as the others.
There are ifs and buts to negotiate as far as quality assessment goes beyond just assigning a numerical score. One of these is that models have meaning; in this study, there was a considerable dispute about whether the player’s current team should be included because many of the data points came from before that player switched teams.
The authors also grouped the statistical teams into two classes based on their statistical expertise. More experienced teams did use more sophisticated methods, but the groups didn’t differ statistically in their likelihood of finding a significant result. However, it would still have been useful to know how well the models described the data.
What to learn from all this?
So you’re reporting a study that hasn’t gone to the expense of finding 29 independent statisticians to try 19 different models; what’s a journalist to do? Here are a few thoughts:
- Look for evidence that multiple models were tried and the reported results were robust to the choice of model. (If multiple models were tried and they only reported the ones with significant results, start getting suspicious).
- Talk to a statistician: don’t just ask them to evaluate the methodology, describe the problem and data, and ask them how they would approach it (get ready for some jargon). Then ask them to review the methods. It’s unlikely that they’ll agree exactly with the paper, but if there is a substantial divergence, there might also be cause to be suspicious. You might need to provide an off-the-record guarantee for the first part, but it can be very helpful to have someone think independently about how to do an analysis, rather than be guided by what has already been done.
- Finally, be aware of how noisy the data is—are the models explaining most of the variability in the data? Are the p-values reported knock-em-out-of-the-park small, or did they just squeak in under a threshold? Noisy data with relatively small effects mean that you need a great deal of data to say anything conclusively and subtle changes in statistical procedures can change the significance of the reported effects.
However, even in this study the statisticians were more consistent than the reported results might suggest; it’s always good to see a second opinion, but a 29th is probably a few more than necessary.
Giles Hooker is Associate Professor of Statistics at Cornell University
Please note that this is a forum for statisticians and mathematicians to critically evaluate the design and statistical methods used in studies. The subjects (products, procedures, treatments, etc.) of the studies being evaluated are neither endorsed nor rejected by Sense About Science USA. We encourage readers to use these articles as a starting point to discuss better study design and statistical analysis. While we strive for factual accuracy in these posts, they should not be considered journalistic works, but rather pieces of academic writing.
Great article, Dr. Hooker! As a social scientist sharing an interest in implicit bias and prejudice, I found this article (and the study itself) quite interesting. I’d like to share a few thoughts and then ask your opinion.
I wonder whether the purpose (and intended audience) of this study relates to its findings (and subsequent conclusions). As you noted, journalists are inherently interested in study results, but may fail to gather potentially significant details about methodology, data, analysis, etc. Consequently, their reports may be somewhat misleading. From an academic (rather than journalistic) perspective, however, I’m curious whether and to what extent the role of theory (and theory development) might have played in the study findings and interpretation. You do mention model quality and raise questions about the relative importance of variables, but did the statisticians let the data drive the theory, or did each team develop a theory and then decide how to test it? Perhaps, the variability of results obtained by the statistician teams may be explained (at least in part) by the variability in their theories (or lack thereof). An alternative comparison, wherein all 29 test the same theory may yield a different pattern of results. What are your thoughts?
Good comment and this is very relevant to the variation in findings. First, I expect that theory is really what lead to the particular data that was collected. There were also discussions about the meanings of the models produced. Almost all teams noted somewhere that these data were not adequate to produce a causal interpretation. There were also long discussions about whether or not a player’s current team should be used, given that many of their data points were from when they played for other teams.
That said, almost all teams took this as a data-driven approach and asked “Can I detect an effect of skin color, accounting for any other possible confounding effects in my data?” That wasn’t universally true — some of the outlying findings were from teams that decided that the simple bivariate relationship (ie not accounting for other covariates) was the right thing to do. That said, I think if you give a statistician a data set and ask “does this covariate affect the outcome”, I think this will be fairly universally understood as “accounting for the effects of any of the other measured covariates”.
Great article, Dr. Hooker! As a social scientist sharing an interest in implicit bias and prejudice, I found this article (and the study itself) quite interesting. I’d like to share a few thoughts and then ask your opinion.
I wonder whether the purpose (and intended audience) of this study relates to its findings (and subsequent conclusions). As you noted, journalists are inherently interested in study results, but may fail to gather potentially significant details about methodology, data, analysis, etc. Consequently, their reports may be somewhat misleading. From an academic (rather than journalistic) perspective, however, I’m curious whether and to what extent the role of theory (and theory development) might have played in the study findings and interpretation. You do mention model quality and raise questions about the relative importance of variables, but did the statisticians let the data drive the theory, or did each team develop a theory and then decide how to test it? Perhaps, the variability of results obtained by the statistician teams may be explained (at least in part) by the variability in their theories (or lack thereof). An alternative comparison, wherein all 29 test the same theory may yield a different pattern of results. What are your thoughts?
Good comment and this is very relevant to the variation in findings. First, I expect that theory is really what lead to the particular data that was collected. There were also discussions about the meanings of the models produced. Almost all teams noted somewhere that these data were not adequate to produce a causal interpretation. There were also long discussions about whether or not a player’s current team should be used, given that many of their data points were from when they played for other teams.
That said, almost all teams took this as a data-driven approach and asked “Can I detect an effect of skin color, accounting for any other possible confounding effects in my data?” That wasn’t universally true — some of the outlying findings were from teams that decided that the simple bivariate relationship (ie not accounting for other covariates) was the right thing to do. That said, I think if you give a statistician a data set and ask “does this covariate affect the outcome”, I think this will be fairly universally understood as “accounting for the effects of any of the other measured covariates”.
The fundamental problem of all of these analyses is that they do not allow us to assess whether skin color influences the probability of a player engaging in a behavior that increases their chance of getting a red card. Consequently, this being a politically loaded question didn’t help in people being upfront about this limitation. It would have been better to also choose an otherwise similar question without this sort of political connotation and compare the results between the two.
The fundamental problem of all of these analyses is that they do not allow us to assess whether skin color influences the probability of a player engaging in a behavior that increases their chance of getting a red card. Consequently, this being a politically loaded question didn’t help in people being upfront about this limitation. It would have been better to also choose an otherwise similar question without this sort of political connotation and compare the results between the two.