The mismeasure of scientific significance
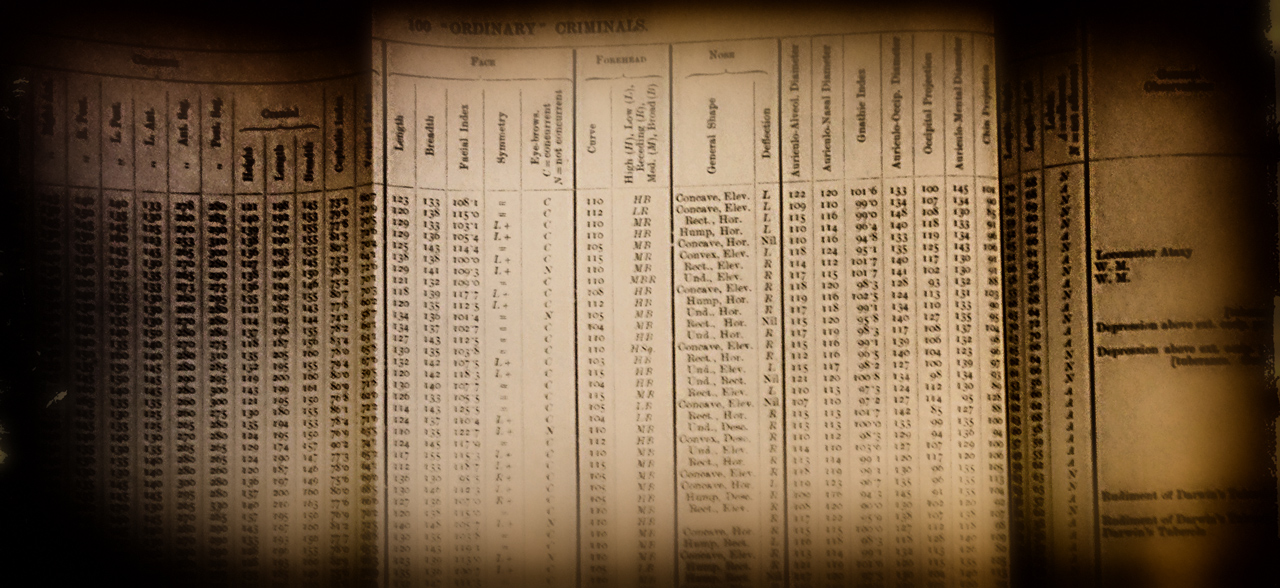
M
arch brings St. Patrick’s Day, the true significance of which has been lost in the froth of green beer and blarney. But without Patrick bringing Christianity to Ireland, Ireland could not have returned it through a rich Insular culture—the written word and the book—to Europe. Now, there is another auspicious moment in the history of learning to add to the month’s calendar of anniversaries: March 7, Significance Day, or—if you will—P Day.
The American Statistical Association—a clerisy for our quantified times—has issued a statement clarifying what a P-value means—or rather doesn’t mean. Indeed, it could be said that by adding up all the things a P-value isn’t you end up with an alarming sense of science in thrall to an absence—P-dolatory—the worship of false significance. As long as your study comparing X and Y ends up with P<0.05, it has found something that is unlikely to be unreal. Science could move forward; your career as an experimentalist had measurable success.
The problem begins with Ronald Alymer Fisher, who, in the 1920s at the Rothamsted Experimental Station in England, laid many of the statistical foundations for designing scientific experiments. Fisher was indubitably brilliant, capable of solving complex mathematical and statistical problems in his head through geometry; but he was sometimes parsimonious when it came to explaining to the less gifted just what those solutions meant or how they might be justified by mathematical proof (it would take years of diligent work by other statisticians to prove, mathematically, why his models worked). The virtue of his landmark book, Experimental Methods for Research Workers, was that you didn’t need a lot of math to use his models to conduct experiments; so too its vice.
As the statistician and science writer Regina Nuzzo notes in a superlative Nature essay on the problem, Fisher intended a P-value to be “an informal way to judge whether evidence was significant in the old fashioned sense: worthy of a second look. The idea was to run an experiment, then see if the results were consistent with what random chance might produce. Researchers would first set up a null hypothesis that they wanted to disprove, such as there being no correlation or no difference between two groups. Next, they would play the devil’s advocate and, assuming that this ‘null hypothesis’ was in fact true, calculate the chance of getting results at least as extreme as what was actually observed. This probability was the P-value. The smaller it was, suggested Fisher, the greater the likelihood that the straw-man null hypothesis was false.”
Unfortunately, as a tool, the P-value became a hammer to a great many experimental nails, and the disputes within statistics—often bitter—over what it actually meant, or whether it meant much at all, were mostly lost on science. The need for ‘evidence’ had found its measure in a rapidly modernizing world; and nothing seemed to succeed in providing publishable evidence quite so much as a P-value smaller than 0.05. Without statistical or mathematical training, statistical significance became a way of foreclosing the difficult task of determining whether a study’s design could actually answer the question a researcher wanted to answer; it was the path of least difficulty in an otherwise highly complex topography of statistical methods, illuminated by software and vouchsafed by academia and scholarly publishing.
The consequence, as Boston University epidemiologist Kenneth Rothman points out in a vigorous essay accompanying the ASA’s statement, is that scientists have “embraced and even avidly pursued meaningless differences solely because they are statistically significant, and have ignored important effects because they failed to pass the screen of statistical significance. These are pernicious problems, and not just in the metaphorical sense. It is a safe bet that people have suffered or died because scientists (and editors, regulators, journalists and others) have used significance tests to interpret results, and have consequently failed to identify the most beneficial courses of action.”
To be fair, statisticians have long been sounding an alarm on P-dolatory in science; but the increasing sense that ‘significance doping’ was behind so many winning results in science—winning results that could not be replicated—spurred the ASA to action; and it is the association’s first time to take a policy position on such a core issue of statistical practice.
“We hoped,” the ASA’s statement reads, “that a statement from the world’s largest professional association of statisticians would open a fresh discussion and draw renewed and vigorous attention to changing the practice of science with regards to the use of statistical inference.” As Ron Wasserstein, ASA executive director, says, the goal is “to steer research into a post P<0.05 era.”
The implications are profound for research, academic publishing, scientific funding, and even the daily journalism of “a new study says”… variety. The statement demands a fundamental rethink in the process of experimental design across many disciplines, and how those designs may be held accountable. As Stanford’s John Ioannidis notes, the real challenge is not simply about getting rid of P-values (for they may yet have some valuable use): it is about creating a scientific culture that embraces “transparency in study design, conduct, and reporting.”
— For the full statement on P-values from the American Statistical Association, click on this link.
P-values made sense when used in the way that Fisher initially proposed:
“If one in twenty does not seem high enough odds, we may, if we prefer it, draw the line at one in fifty (the 2 per cent point), or one in a hundred (the 1 per cent point). Personally, the writer prefers to set a low standard of significance at the 5 per cent point, and ignore entirely all results which fail to reach this level. A scientific fact should be regarded as experimentally established only if a properly designed experiment rarely fails to give this level of significance.”
[Fisher RA (1926), “The Arrangement of Field Experiments,” Journal of the Ministry of Agriculture of Great Britain, 33, 503-513.]
Later workers, and to an extent Fisher himself in later work, forgot or ignored the “rarely fails” bit. See the selection of Fisher quotes at: http://www.jerrydallal.com/lhsp/p05.htm . P-values were treated as absolute measures of the weight of evidence rather than as measures of the shift of evidence. Regina Nuzzo, in the Nature essay that was quoted, explains this well.
What may be the larger problem arises from the large-scale neglect of the experimental design understandings that Fisher pioneered. In agricultural research, largely as a result of Fisher’s influence, it became standard practice for researchers to work closely with experimental design experts. Failure to follow that lead, especially in laboratory science, has had disastrous consequences for the reproducibility of published studies. Research scientists in those areas urgently need to start working closely with experimental design experts. Problem is, that area of expertise has been too much neglected in the rush for a theoretical novelty that is often far removed from the practicalities of the lab.
Thank you John for that valuable comment!
Trevor
Jct: So he suggested outside two standard deviations in the Bell Curve (1/20) was significant. So set it to three standard deviations (1/700). Or less. No major sin to say 20:1 odds against warrant investigation.
P-values made sense when used in the way that Fisher initially proposed:
“If one in twenty does not seem high enough odds, we may, if we prefer it, draw the line at one in fifty (the 2 per cent point), or one in a hundred (the 1 per cent point). Personally, the writer prefers to set a low standard of significance at the 5 per cent point, and ignore entirely all results which fail to reach this level. A scientific fact should be regarded as experimentally established only if a properly designed experiment rarely fails to give this level of significance.”
[Fisher RA (1926), “The Arrangement of Field Experiments,” Journal of the Ministry of Agriculture of Great Britain, 33, 503-513.]
Later workers, and to an extent Fisher himself in later work, forgot or ignored the “rarely fails” bit. See the selection of Fisher quotes at: http://www.jerrydallal.com/lhsp/p05.htm . P-values were treated as absolute measures of the weight of evidence rather than as measures of the shift of evidence. Regina Nuzzo, in the Nature essay that was quoted, explains this well.
What may be the larger problem arises from the large-scale neglect of the experimental design understandings that Fisher pioneered. In agricultural research, largely as a result of Fisher’s influence, it became standard practice for researchers to work closely with experimental design experts. Failure to follow that lead, especially in laboratory science, has had disastrous consequences for the reproducibility of published studies. Research scientists in those areas urgently need to start working closely with experimental design experts. Problem is, that area of expertise has been too much neglected in the rush for a theoretical novelty that is often far removed from the practicalities of the lab.
Thank you John for that valuable comment!
Trevor
Jct: So he suggested outside two standard deviations in the Bell Curve (1/20) was significant. So set it to three standard deviations (1/700). Or less. No major sin to say 20:1 odds against warrant investigation.
What are some examples of important relationships that failed to reach statistical significance in a study with sufficient power?
What are some examples of important relationships that failed to reach statistical significance in a study with sufficient power?
Psychologist J. Philippe Rushton accused Gould of “scholarly malfeasance” for misrepresenting and for ignoring contemporary scientific research pertinent to the subject of his book, and for attacking dead hypotheses and methods of research . He faulted
Psychologist J. Philippe Rushton accused Gould of “scholarly malfeasance” for misrepresenting and for ignoring contemporary scientific research pertinent to the subject of his book, and for attacking dead hypotheses and methods of research . He faulted