Causation vs Correlation
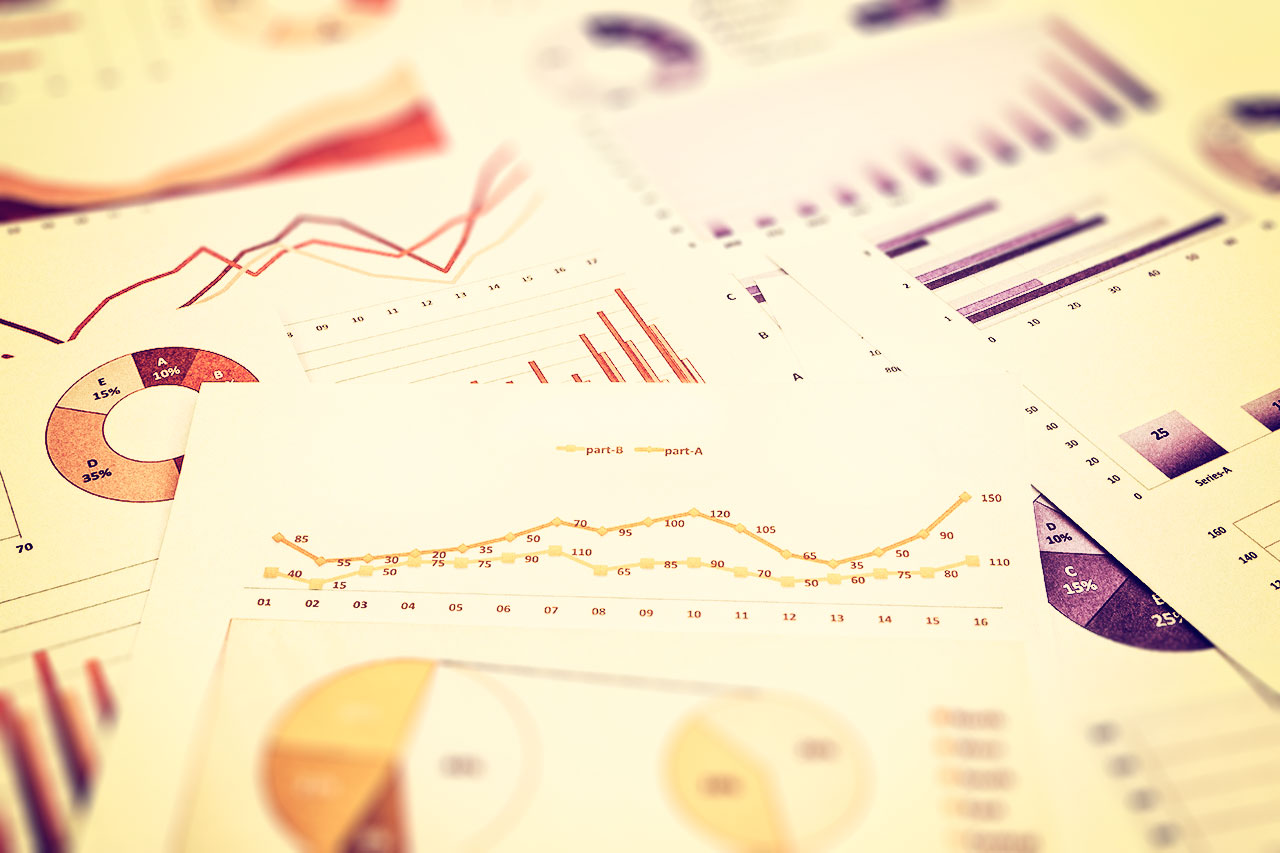
Unfortunately, intuition can lead us astray when it comes to distinguishing between the two. For example, eating breakfast has long been correlated with success in school for elementary school children. It would be easy to conclude that eating breakfast causes students to be better learners. Is this a causal relationship—does breakfast by itself create better students? Or is it only a correlation: perhaps not having breakfast correlates highly with other challenges in kids’ lives that make them poorer students, such as less educated parents, worse socio-economic status, less focus on school at home, and lower expectations.
It turns out that kids who don’t eat breakfast are also more likely to be absent or tardy—and absenteeism plays a significant role in their poor performance. This may lead one to believe that there is not a causal relationship. Yet breakfast may encourage kids to come to school (and on-time), which then improves their performance in school, and so perhaps encourages attendance, which then results in better performance. In a recent literature review, there were mixed results suggesting that the advantages of breakfast depend on the population, the type of breakfast provided, and the measurement of “benefit” for the kids. Breakfast seems to have an overall positive impact on cognitive performance, especially memory tasks and focus. Not surprisingly, the benefit seems greater for kids who are undernourished. But the clear message here is that a causal relationship has been extremely hard to establish, and remains in question.
Many studies are designed to test a correlation, but cannot possibly lead us to a causal conclusion; and yet, obvious “reasons” for the correlation abound, tempting us toward a potentially incorrect conclusion. People learn of a study showing that “girls who watch soap operas are more likely to have eating disorders”— a correlation between soap opera watching and eating disorders—but then they incorrectly conclude that watching soap operas gives girls eating disorders. It is entirely possible that girls who are prone to eating disorders are also attracted to soap operas.
There are several reasons why common sense conclusions about cause and effect might be wrong. Correlated occurrences may be due to a common cause. For example, the fact that red hair is correlated with blue eyes stems from a common genetic specification that codes for both. A correlation may also be observed when there is causality behind it—for example, it is well established that cigarette smoking not only correlates with lung cancer but actually causes it. But in order to establish cause, we have to rule out the possibility that smokers are more likely to live in urban areas, where there is more pollution—and any other possible explanation for the observed correlation.
In many cases, it seems obvious that one action causes another; however, there are also many cases when it is not so clear (except perhaps to the already-convinced observer). In the case of soap-opera watching anorexics, we can neither exclude nor embrace the hypothesis that the television is a cause of the problem—additional research would be needed to make a convincing argument for causality. Another hypothesis might be that girls inclined to suffer poor body image are drawn to soap operas on television because it satisfies some need related to their poor body image. Or it could be that neither causes the other, but rather there is a common trait—say, an overemphasis on appearance in the girls’ environment—that causes both an interest in soap operas and an inclination to develop eating disorders. None of these hypotheses are tested in a study that simply asks who is watching soaps and who is developing eating disorders, and finding a correlation between the two.
How, then, does one ever establish causality? This is one of the most daunting challenges of public health professionals and pharmaceutical companies. The most effective way of doing this is through a controlled study. In a controlled study, two groups of people who are comparable in almost every way are given two different sets of experiences (such one group watching soap operas and the other game shows), and the outcome is compared. If the two groups have substantially different outcomes, then the different experiences may have caused the different outcome.
There are obvious ethical limits to controlled studies: it would be problematic to take two comparable groups and make one smoke while denying cigarettes to the other in order to see if cigarette smoking really causes lung cancer. This is why epidemiological (or observational) studies are so important. These are studies in which large groups of people are followed over time, and their behavior and outcome is also observed. In these studies, it is extremely difficult (though sometimes still possible) to tease out cause and effect, versus a mere correlation.
Typically, one can only establish a causal relationship if the effects are extremely notable and there is no reasonable explanation that challenges causality. This was the case with cigarette smoking, for example. At the time that scientists, industry trade groups, activists and individuals were debating whether the observed correlation between heavy cigarette smoking and lung cancer was causal or not, many other hypotheses were considered (such as sleep deprivation or excessive drinking) and each one dismissed as insufficiently describing the data. It is now a widespread belief among scientists and health professionals that smoking does indeed cause lung cancer.
When the stakes are high, people are much more likely to jump to causal conclusions. This seems to be doubly true when it comes to public suspicion about chemicals and environmental pollution. There has been a lot of publicity over the purported relationship between autism and vaccinations, for example. As vaccination rates went up across the United States, so did autism. And if you splice the data in just the right way, it looks like some kids with autism have had more vaccinations. However, this correlation (which has led many to conclude that vaccination causes autism) has been widely dismissed by public health experts. The rise in autism rates is likely to do with increased awareness and diagnosis, or one of many other possible factors that have changed over the past 50 years.
Language further contorts the distinction, as some media outlets use words that imply causality without saying it. A recent example in Oklahoma occurred when its Governor, Mary Fallin, said there was a “direct correlation” between a recent increase in earthquakes and wastewater disposal wells. She would have liked to say that the wells caused the earthquakes, but the research only shows a correlation. Rather than misspeak, she embellished “correlation” with “direct” so that it sounds causal.
At times, a correlation does not have a clear explanation, and at other times we fill in the explanation. A recent news story reports that housing prices in D.C. correlate with reading proficiency. Many stories can be crafted to explain the phenomenon, but most people would be reluctant to conclude that a child’s reading proficiency could cause the price of their house to be higher or lower, or vice-versa. In contrast, a news story reporting that “30 years of research found a positive correlation between family involvement and a student’s academic success” in Florida feels like it has the weight of causality. The big difference between these two different correlations is our own belief in a likely mechanism for family to contribute to better grades.
In general, we should all be wary of our own bias: we like explanations. The media often concludes a causal relationship among correlated observances when causality was not even considered by the study itself. Without clear reasons to accept causality, we should only accept the existence of a correlation. Two events occurring in close proximity does not imply that one caused the other, even if it seems to makes perfect sense.
I discovered your stat stories this morning while poking around the internet looking for good examples of bad statistics. I am still reading your stories with relish an hour after starting – they are interesting, fun, thought provoking and at a level I can use in my intro stat classes this fall. Thank you for these gems – I am planning to put them to good use this term.
Thank you Margaret: We have big expansion plans in the next year!
Great!!!!! Very informative and the style of presentation was extremely beautiful
I’m a high school student in a college level Stats course and reading this has been so interesting. I’m starting to think about a career in Stats
Good for you Zachary! The world needs more statisticians! And it’s willing to pay too!
See what you’ve caused!?
This was a great source of information. I’m currently writing an essay for extra credit and this is the first clear explanation I have found that truly approaches the topic the way I need. Thank you so much.
Dr. Goldin, great piece. Is there a point where the size, scope or length of time scale of the data begins to demonstrate such an overwhelming correlation that cause and effect is much more certain? I’m thinking about this in the context of business, where most of the decisions we make each day are based on correlation v. absolute certainty re cause and effect. It seems logical, for example, that correlations in larger bodies of data over longer periods of time would be inherently more reliable and potential more indicative of cause and effect than two quarters of data. Your thoughts would be greatly appreciated. Thanks!
Most readers won’t know the difference between causation and correlation. In fact, many reporters who use those two words don’t fully understand either. However, what would be the proper way to explain that not all smokers suffer from lung cancer?
By doing a controlled experiment
x causes y if the lagged values of x improve the predectibilty of the current value of y
Thank you for your help, the clearest example I’ve seen! I’m currently working on my dissertation and this really helped! Thanks
Thank you for your help, the clearest example I’ve seen! I’m currently working on my dissertation and this really helped! Thanks
go westfield, urban ed!!!
go westfield, urban ed!!!
“If one action causes another, then they are most certainly correlated. “. Could you please elaborate a bit more on that, i.e. can one action cause another, and not be correlated?
Right away I am ready to do my breakfast, when having my breakfast coming over again to read more news.
Great!
Good!
Very insightful. Your article will change the way I discuss Causation vs Correlation. Thank you!
Thanks for finally talking about >Causation vs
Correlation <Loved it!
I know I’m late to the game here, and your post is older, but I thought I’d mention that the reporting on the opioid crisis (and its correlation to prescriptions of opioids to pain patients) is a perfect example of this. In a few years, we will realize that there is a correlation between the two, but not causation, and 99.9% of the world has been misled because they don’t know the difference, and the government has a different agenda than presenting the facts in a way that will lead to good policy. Opioid prescribing has been decreasing since 2010, when ODs have skyrocketed.
A member of The Alliance for Intractable Pain explains it the best: “The US CDC publishes a lot of data on opioid prescribing by physicians and on overdose-related deaths. But they’ve never bothered to put the two together. When one does this exercise, we learn some startling things. Chief among them is that there is no relationship between State-by-State rates of opioid prescribing by doctors, versus rates of overdose-related mortality. NONE. The chart for 2016 is a shotgun pattern without trend lines or correlation. The contribution of medically managed opioid prescriptions to opioid mortality is so small that it gets lost in the noise of illegal street drugs. And this includes both prescriptions used by patients, and pills diverted by theft or being given to an under-insured relative. We also learn from the CDC data that the demographics of chronic pain and of overdose mortality are almost entirely different. The “over-prescribing” narrative doesn’t work and never did. If prescribing was contributing to overdose deaths, we would expect to see higher mortality in age groups that receive more prescriptions. But there is no such trend. In the past 17 years, death rates in youth and young adults have skyrocketed while opioid mortality in people over age 50 has remained stable at the lowest levels for any age group. Moreover, the typical initiating substance abuser and the typical chronic pain patient are greatly different people. The typical abuser is a young male who has never seen a doctor, and who may have a history of mental health issues and family stress. The typical chronic pain patient is a woman in her 40s or older. If her life is stable enough to see a doctor regularly, she will almost never be a substance abuser. These trends are summarized and supported with graphics both on ACSH and in an article in the June 21 edition of The Crime Report, titled “The Phony War Against Opioids – Some Inconvenient Truths”. Readers can google the title to find the article.” https://www.acsh.org/news/2018/11/05/who-telling-truth-about-prescription-opioid-deaths-dea-cdc-neither-13569. http://www.atipusa.org
I never wanted to learn about these things until I became a chronic pain patient threatened with living my life in agony. But I wish journalists would read your article and learn about this too. Thanks….
Excellent