African American Boys and Autism
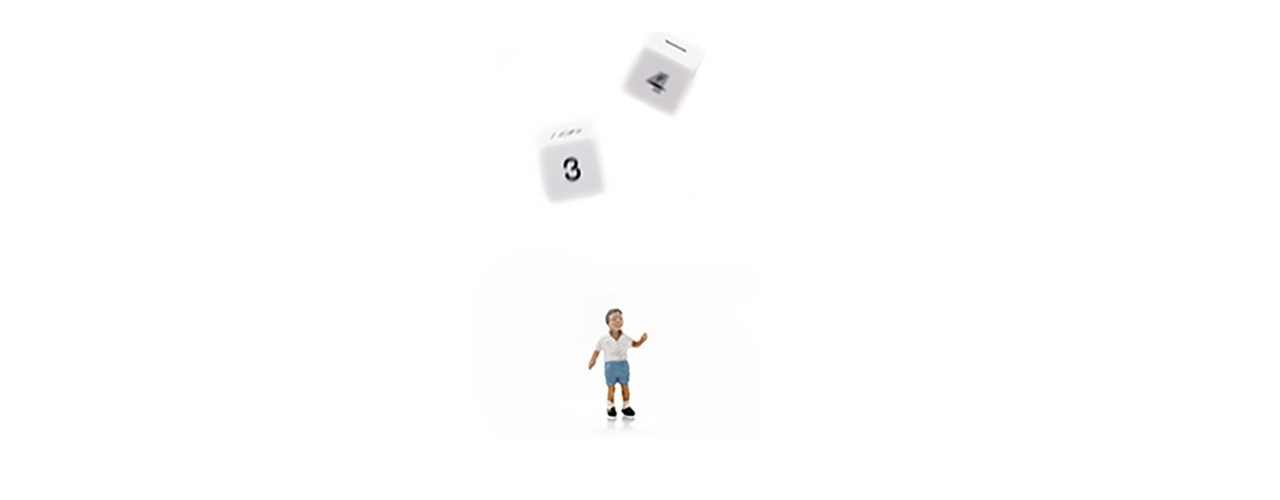
A shocking reanalysis of CDC data claimed that African American boys had a greater risk of autism associated with the time they were vaccinated, and that the agency had covered up this finding. The study ignited a wave of conspiracy charges across the web; but did its author understand how to do statistical analysis?
In 2004, Dr. Frank DeStefano et al. from the Centers for Disease Control (CDC) published a case-control study that compared the vaccine records of autistic kids to the vaccine records of non-autistic kids in a population of children in Atlanta, Georgia. The study found no link between autism and the timing of the MMR vaccine. DeStefano’s paper, and several others pointing to the lack of evidence of a relationship between autism and vaccination, were coauthored by William Thompson, another CDC vaccination expert.
Recently, Thompson shared CDC data from this study with Brian Hooker, who has a PhD in biochemical engineering, and who appears to hold deep convictions about the relationship of autism to vaccination. Hooker reanalyzed the data, included some original data that had not been included in the CDC analysis, and found a large effect for African American boys that had not previously been reported.
This effect—a 3.4-fold increase in the risk of autism—sent shockwaves through the anti-vaccination movement this summer, which were amplified by a YouTube video claiming evidence of a CDC cover up.
But Hooker, who appears to have had little training in statistical analysis made errors that can really skew the statistical picture, making it look like associations were present that may well be spurious. Perhaps this is why Hooker’s paper was retracted. But a retraction of a paper does not prove its falsehood, and a closer look may shed some light on the critical importance of study design.
First of all, Hooker chose to look at African American boys because he already knew there was an association in his sample. Though this might, intuitively, seem like the obvious and the right thing to do, it isn’t. In fact, it’s a basic error in statistical analysis. If you first look at your data and choose the most extreme subgroup among all the data you have, you are bound to find statistical correlations that do not exist in the whole population. This phenomenon is the reason why a study’s protocol is designed before the data are collected; to do so after the data have been collected is tantamount to cherry picking.
Cherry picking aside, big questions remain about what exactly Hooker did (statistically speaking) in his paper. Let’s say, for the sake of argument, that the protocol had been designed to consider African American kids as a separate group before any data was collected. Did Hooker try to calculate the relative risk of autism, depending on vaccination status? Unfortunately, using DeStefano’s data, he simply couldn’t have.
To explain why the relative risk of autism could not have been calculated with the CDC data, we take a brief but relevant foray into conditional probability: DeStefano et al. found that among autistic kids, vaccination rates were similar to those among kids who did not have autism.
This is a distinctly different from the question of whether, among vaccinated kids, there is a higher rate of autism.
In the language of conditional probability, if A is the condition that someone has autism, and B is the condition that someone was vaccinated early, then DeStefano et al. evaluated the probability that they were vaccinated early given they have autism. This is rendered in mathematical notation as Pr(B |A), where the | stands for “given”.
DeStefano compared this conditional probability—the probability that they were vaccinated early given they do not have autism, which is denoted as Pr(B | not-A). This is distinct from finding the probability of autism among vaccinated children, or Pr(A | B).
There is a way to get from Pr(B | A) to Pr(A | B) – if you know the overall probabilities. Bayes’ Theorem says that
Pr(A|B) = Pr(B|A) * P(A) / Pr(B)
So if you know the probability of vaccination Pr(B) and the probability of autism Pr(A), then you can go from the probability of vaccination given autism to the probability of autism given vaccination.
Why is this important? Because it points to two fatal mistakes Hooker made in the attempt to disavow DeStefano et al.
In his introduction, Hooker describes the work by DeStefano et al. in these terms: “the study authors assessed relative risk for obtaining an autism diagnosis for those children receiving the first MMR vaccine before and after 18 months, 24 months and 36 months of age. Destefano [sic.] et al. found a statistically significant relative risk of 1.49 (95% confidence interval [CI]: 1.04 – 2.14) at the 36 month cut-off (i.e., in a comparison of children receiving the MMR before versus after 36 months).”
In this account, it would seem that DeStefano et al. found that kids who received the MMR vaccine before 36 months had a higher risk of autism compared to kids who received it after 36 months.
But DeStefano et al. were not calculating the risk of autism given vaccination; they found the rate of vaccination given autism. Hooker implies that children who receive the vaccination are more likely to get autism. But DeStefano showed that children who have autism are more likely to get vaccinated.
There’s another subtle point, which reveals Hooker’s lack of expertise in statistics: DeStefano et al. were speaking about an odds ratio while Hooker was speaking about relative risk. Now both odds ratio and relative risk trend in the same direction—if they are over the value 1.0, they each imply that one group has more risk than the other. But odds ratios and relative risks tell a very different story; here’s a nice example as to how.
In DeStefano et al.’s data, the rate of vaccination among autistic kids at age 36 months was 93.4 percent and the rate of vaccination among kids who did not have autism was 90.6 percent. The odds ratio is 1.49, and it was statistically significant.
On the other hand, the “relative risk” of vaccination given autism is 1.03, compared to the “risk” of vaccination among kids without autism. In other words, kids who have autism are 3 percent more likely to get vaccinated.
This is a long way from the outrage produced by Hooker’s confusing statement suggesting 36-month old babies have a 49 percent increased chance of autism if they are vaccinated with MMR before that point. Nope. Autistic kids have a higher rate of vaccination. According to DeStefano, this higher rate of vaccination results from parents’ seeking medical care and educational services for children with autism.
But what about Hooker’s hook?
The biggest statistical challenge of the autism/vaccination debate is deciding which data can be compared. It would be so ideal if we could simply corral a bunch of kids from birth, have them lead completely equivalent lives, and give only half of them vaccinations, giving the other kids just saline or no injections at all. Then we can watch and wait: are the vaccinated kids more likely to develop autism? Obviously such an experiment would be unethical for multiple reasons, so instead, our main tool is to consider the question from an observational point of view: design a study, collect data, and analyze said data.
Hooker maintains that DeStefano didn’t use all the data he collected, and that, had that missing data been included, a much stronger relationship between vaccination and autism can be seen. In particular, Hooker’s retracted article claims to have shown that African American boys do have a higher risk of autism if they are vaccinated before 36 months. And Hooker used the DeStefano data to show it, or so he claims.
While it may seem of little statistical import, the design of the DeStefano study is essential and determines the kinds of results one can obtain from the data collected. The DeStefano study is a case-control study, which means that kids with autism were included (the cases) and then a separate set of kids without autism (the controls) were matched by various features to be similar to the ones with autism, except that they aren’t autistic.
A key element of a case-control study is that the controls are chosen to be similar to the cases in any way that could otherwise bias the results due to selection bias. For example, if you wanted to see if there’s a link between candy and cavities, you might compare those who get cavities (cases) to those who don’t (controls) and see whether those who get cavities eat more candy. But you would want to make sure both groups have similar brushing habits and dental insurance, otherwise the candy might be unfairly blamed if in fact the difference in cavity levels is entirely due to poor brushing habits.
A case-control study allows you to compare the rates of exposure to a possibly causal factor for autism; for example, one can explore whether kids who have autism are more likely to have been vaccinated than kids who do not have autism. But it cannot tell you how prevalent autism is among people who get vaccinations. That’s because you start by having cases, rather than observing how those cases might come about in a large population. The case-control design is especially useful if only a small percentage of the population could be designated as a “case”. According to the CDC, approximately 1 to 2 percent of all U.S. children have an autism spectrum disorder.
Yet Hooker did something funky with the data: he pulled out the African American kids, and did an analysis on them. One problem with pulling out a subgroup from a case-control study is that race was not directly controlled for in the DeStefano study. DeStefano included 624 kids with ASD, and 1824 controls, matched by their school, their birth year, and their sex.
The first question one might ask is whether the African American controls were still controls – it would be easy to accidentally introduce bias into the controls since they are no longer truly controls for the sample of cases chosen.
Secondly, instead of emulating the case-control design of DeStefano et al., he compares rates of autism among kids who have had (or not had) vaccines at certain ages. He compared the rate of autism among kids who had their first MMR before 18 months, to the rate among kids who had their first MMR after 18 months. Then he did the same analysis for 24 months and 36 months. (Well, at least in one section of his paper, he seems to believe he’s done this; in another section, he implies he is looking at vaccination rates among autistic versus non-autistic kids, which is the best he could do with his data.)
What’s wrong with this idea of taking case-control data and doing a different analysis? Again, the problem lies in how the data are selected. Hooker’s analysis would make sense if the data were obtained in the context of a cohort study. In a cohort study, one chooses first a population that has some exposure to a possibly dangerous substance (say, vaccination), and then one waits to see who develops the disease (in this case, ASD).
The essential part of a cohort study is that the control group should not be exposed to vaccination, yet should otherwise be similar. Again, the rate of autism among those not exposed to vaccination is recorded. Finally, one compares the rate of autism development in both groups. But the cases Hooker uses did not occur randomly among a group of people exposed to vaccination—they were chosen because they were cases. In particular, there was no attempt in the choice of cases to make them representative of all kids who get autism in a specific population. This means that they may—or may not—represent the kids who would have developed autism had a group of children (some vaccinated and some not) been observed over time.
Finally, there is something strange about how Hooker’s data emerge. Remember that Hooker mistakenly spoke about odds ratios as if they were relative risk when describing the results in the DeStefano et al. study. He reports having rerun those analyses and obtained the exact same numbers that were DeStefano’s odds ratios for the total group, with the exact same confidence intervals. It’s highly improbable that a relative risk analysis of the likelihood of autism given vaccine versus the likelihood if not given vaccine, ended up with the exact same proportion as the odds ratio of vaccination given autism compared to vaccinated given no autism, with the exact same confidence interval. That seems almost too good to be true, and it is. We’re left with wondering exactly what calculation is reported in Hooker’s paper when it comes to African American boys.
The Hooker paper shows the damage that can be done when a study is incorrectly designed to answer a question, and then it incorrectly analyses the data it produces. These flaws will be obvious to statisticians and to scientists who understand statistical analysis. The problem is how to undo the damage among a public that is skeptical of scientific authority, and is suspicious or even hostile toward vaccination.
Please note that this is a forum for statisticians and mathematicians to critically evaluate the design and statistical methods used in studies. The subjects (products, procedures, treatments, etc.) of the studies being evaluated are neither endorsed nor rejected by Sense About Science USA. We encourage readers to use these articles as a starting point to discuss better study design and statistical analysis. While we strive for factual accuracy in these posts, they should not be considered journalistic works, but rather pieces of academic writing.
This is a great explanation. Very clear. Thanks!
It seems to me that EVEN if the whole analysis were more rigorous, the fact that this is an exploratory study invalidates any conclusive finding. At it’s best, this kind of research can only indicate new questions to ask in a subsequent (hopefully preregistered) confirmatory study.
I am amazed at the power of statistics. Statistics have such influence to shape or perceptions, beliefs, and behavior. We must be more discerning before we accept something as fact as we have learned that how data is analyzed can literally change the outcome of what is proposed as fact.
You may be right about the Hooker paper, but still, whatever Hooker did wrong or right, that was no reason to retract the paper– a retraction which was against the stated retraction policy of the publisher. I asked the publisher why Hooker’s paper was retracted when it violated none of their policies and no reply was offered. The paper should have stood: any objections, such as yours, could have been posted as comments on the paper. Furthermore, you miss an important point, and that is that DeStefano et al deliberately removed data they felt would have shown that some children were more likely to get autism after vaccination. The point is not whether the data really did show that, but rather the point is that DeStefano et al, at least according to Dr. Thompson (the CDC whistleblower involved in this paper) believed that it would, and worked to remove it. So that leads to another more important question that your analysis, even if correct, glosses over: how many more studies were impacted by this sort of bias not to present evidence that vaccines may be causing more harm than we believe?
To answer this, you may want to look at Ball’s 2001 paper on thimerosal (“An Assessment of Thimerosal Use in Childhood Vaccines”) even though this concerns a toxicological question more than a mathematical one, but still you might want to read this and ask, is there any bias here? Are the facts presented fairly? Specifically, did children really get “mostly safe doses” (to paraphrase) of ethylmercury in the 1990s? You may have some trouble with this so here’s a hint: if a person takes too much aspirin and has to go to the emergency room, does the doctor average the dose received over six months? If you think about it this way you’ll see a clear bias– or more accurately, a distortion of the evidence, a type of distortion that ignored mercury levels and prompted officials not to have children tested for mercury levels received from vaccination. What would you have done if you’d known that children received many times over safe doses of ethylmercury in the 1990’s? I hope you would have recommended that all children be tested for mercury exposure, just to be safe. But that isn’t what happened.
You are right it’s not about the accuracy. It’s about the refusal to go deeper because it threatens their reputation and bottom line.
Don ~
Do you seriously think that a paper in which the data were not evaluated properly should not be retracted? That makes no sense at all.
Another reason Hooker’s paper was retracted is that he failed to disclose a major conflict of interest: his child’s claim pending in the Office of Special Masters of the U.S. Court of Federal Claims, which administers a no-fault system for litigating vaccine injury claims. Hooker should never have tried to do a study himself. As Dr. Goldin shows us, he did not know how to do it. If he had really been interested in showing that the CDC data showed an increase in autism he should have arranged for an impartial third party to look at it. The CDC actually did that, and the conclusions of the original authors were upheld.
The metabolism of ethylmercury after its administration to infants in vaccines has been studied. Vaccines do not raise blood mercury levels. Any contribution to body mercury levels from vaccines is overwhelmed by other sources of mercury, in particular methyl mercury in the diet. The fact that the thimerosal is injected does not make a difference. It is picked up from the muscle and makes its way to the bloodstream, just as ingested mercury makes its way from the gut. It is inappropriate to compare methyl mercury and ethyl mercury, too. Their effects are very different, just as the effects of methyl alcohol and ethyl alcohol are very different. Those who claim that the amount of mercury in vaccines was “many times over safe doses of ethylmercury” are basing that claim on the amounts of mercury in IV nutrition of newborns, and that comparison is not valid since the dose in vaccines will not be anywhere near the dose possibly given in such fluids. Finally, the incidence of autism has not declined since thimerosal was removed from vaccines. That in itself demonstrates that thimerosal does not cause autism.
By the way, Hooker failed to prove his claim in vaccine court. His child’s medical records clearly showed there were signs of autism before the vaccine was administered that Hooker claimed caused the problem. Despite his PhD, Hooker is no more able to get past the ergo hoc post propter hoc fallacy than thousands of less scientifically sophisticated parents of children with autism.
Martha~
“Finally, the incidence of autism has not declined since thimerosal was removed from vaccines. That in itself demonstrates that thimerosal does not cause autism.”
This touches on an area I’m interested in. Can you give me any studies that support this please?