While there is plenty of evidence that chocolate consumption can be both beneficial and detrimental to your health, have you ever considered that it may make you a Nobel Laureate? Likely not, but there are some bizarre and improbable correlations that show two unlikely variables have a positive relationship. How do scientists then know when a relationship they find in the laboratory is factual?
Let’s break this down.
Scientific studies aim to prove something. That something is typically an unknown or undefined relationship between two previously functionally-distinct phenomena. For example, insulin insensitivity may lead to amyloid-beta plaques in the brain (a common feature of Alzheimer’s). So then, is Alzheimer’s really a form of Diabetes Type III?
According to research done by Dr. Suzanne De La Monte at Brown University, there is evidence that Alzheimer’s may be just another form of diabetes. Describing novel therapeutic targets in the human body (such as insulin in the brain) relies on a researcher’s ability to draw “lines” and connect the dots; hence, proving that one facet can influence another. These lines can be fuzzy dotted lines—if experimental evidence is weak or suggestive—or they could be firm, solid lines that clearly show a direct causal relationship between the two variables.
The difference between correlation and causation has misled countless observers in science and other professions.
Correlation is a mutual relationship, where two or more attributes show a tendency to trend together; however, it is not firm proof that those two variables are linked to one another, nor does it establish that one influenced the other. One example might have been the aforementioned bond between chocolate consumption and Nobel Laureates. Mathematicians would argue that while this relationship is unlikely, there is another unknown variable or sequence of interconnected variables that somehow connects the two.
Another strange correlation that was published in the 1930s connected the influx of storks into Copenhagen with an increase in the human birth rate there following World War II.
Everyone knows that storks do not deliver babies—most of us remember being subjected to the awkward coming-of-age where-do-babies-come-from discussion. How then did this correlation between storks and babies come about?
Scientists speculate that after World War II, Copenhagen’s population grew through an increase in migrants from rural lands and a post-war baby boom. Consequently, as the population of the city increased, there was a need for more city housing so new buildings were constructed to accommodate the growing number of people. These new housing structures were inviting nesting places for migrating storks; thus, storks increased in Copenhagen. Therefore, stating that an increase in storks leads to an increase in births would be as preposterous as saying margarine consumption causes divorce in the U.S. state of Maine—which would appear to be true to the untrained eye.
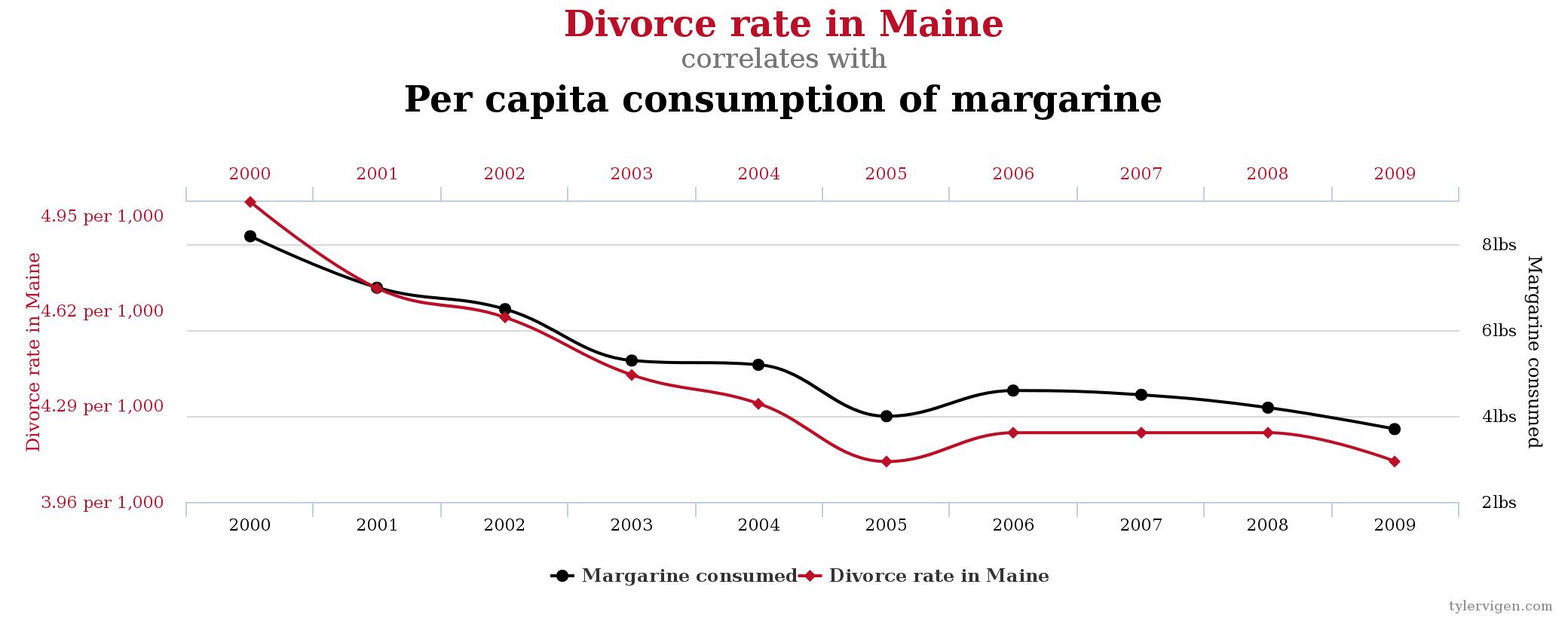
Now that we have laid down some groundwork for correlation, how does it differ from causation?
Causation is when one action produces an effect, result or condition, such that one variable is dependent or influenced (either directly or indirectly) by another. This is what scientists strive to achieve in their scientific research: to show a positive or negative effect (with the polarity of the observation dependent on the scientific question) between two otherwise unlinked features.
In order to validate their observations, scientists use a plethora of experiments in an attempt to gather sufficient evidence to confirm or refute their hypothesis. The strength and reproducibility of that effect may be dictated by the types of experiments and techniques used by scientists. This is why designing the study to achieve the results that clearly show a causal relationship is necessary to properly “prove” the end result.
One area of research where confusion between correlation and causation can lead to disastrous outcomes is in the realm of clinical trials.
Clinical trials rely on sound statistical confirmation that the new drug under testing reveals positive effects in patients. In order for clinicians to prove that the novel therapeutic agent is causing the desired results and not simply eliciting them by chance or by correlation, researchers have to carefully consider the design of the study. It is for this reason that clinicians select patients with features that minimize the likelihood of convoluting the outcome.
For example, if you hope to show that your drug cures blindness, you will likely select patients who are not seeking alternative treatments to restore sight (that might interfere with your study and claim credibility for your drug’s success). Similarly, if you are testing a drug that targets to reduce atherosclerosis (a hardening and narrowing of the arteries), you may choose to exclude patients predisposed to heart complications. This would help ensure your drug is not held responsible for toxic, adverse side effects.
To aid in the proper interpretation of whether the drug is effective or not, and whether that effect is “real”, scientists need to statistically prove their results while simultaneously minimizing any convoluting influences. This is why mere correlations are not sufficient to prove anything. They might have statistical significance, but it does not mean that is a reliable and reproducible relationship (since there may be additional and sometimes unaccounted, convoluting influencers).
Last year, Professor Brian Nosek of University of Virginia and his team published that upon reviewing 100 psychology studies (where 97 percent reported statistical significance), only 36 percent of those results, when repeated, were still significant. In short, this means that many experiments are contextual; there may be factors impacting the initial study that are not captured in the original report—take the stork example, where there was no mention of the migrant influx. Without having all these factors identified and shared, then chances of obtaining the same results fall dramatically when fellow scientists attempt to replicate the original results.
Correlation does not imply causality, and as a consumer of public media and journalism, you should be sure to ask yourself whether the study under discussion has solid statistics and openly states caveats, such as potential convoluting factors.
Next time you read about a new medicine, determine for yourself whether the first variable, such as a new drug, truly causes an effect on the second attribute, such as efficacy or side effects. Be ready to identify when evidence is not sufficient to validate the claims. Be skeptical, dig deeper and seek out the scientific literature.
In the words of Merovingian (sometimes known as the Frenchman) in “Matrix Reloaded”:
“You see there is only one constant. One universal. It is the only real truth. Causality. Action, reaction. Cause and effect.”
Follow that critical thought process and you may be on your way to becoming the next Nobel Prize winner.
— If you are interested in more strange and unlikely correlations, be sure to check out the relationships between consuming organic foods and autism, as well as my personal favorite: the connection between pirates and global warming.