Odds or Probability?
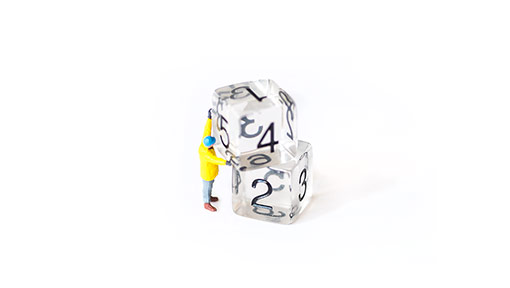
What are the odds you know the difference between odds and probability? While not the same, the two words often are conflated in lay discussions and, perhaps, most ironically, in a recent New York Times article, How Not to Be Fooled by Odds. Numerous times, the article used the word “odds” when it should have used the word “probability.” For example, the very first line states, “The Upshot puts odds of a Republican takeover of the Senate at 74 percent.”
To quickly illustrate the difference between the two terms, the odds in favor of heads in a standard coin flip is one to one (in mathematical notation, 1:1). The probability of heads is 50 percent. The Times should have said either
- “The Upshot puts the probability of a Republican takeover of the Senate at 74 percent;” or
- “The Upshot puts the odds of a Republican takeover of the Senate at about 3 to 1.
The Ask Dr. Math forum has several entries on odds versus probability. Summarizing, one way to conceptualize (non-technically) the probability of an event is the number of ways that an event can occur divided by the total number of possible outcomes. The probability of heads in a fair coin flip is 1/2 (50 percent). The probability of drawing a red card from a standard deck of cards is 26/52 (50 percent). The probability of drawing a club from that deck is 13/52 (25 percent). And so on.
The odds for an event is the ratio of the number of ways the event can occur to the number of ways it does not occur. For example, using the same events as above, the odds for:
- the coin flipping heads is 1:1 (said “1 to 1”)
- drawing a red card from a standard deck of cards is 1:1; and
- drawing a club from that deck is 1:3.
Probabilities should also be stated in the range zero to 100 percent (or the decimal or fractional equivalent). Odds are typically expressed as two integers.
Another common misuse of the word “odds” is on lottery websites. Powerball makes this mistake, but, interestingly, Megamillions does not.
Understanding the distinction between odds and probability is not just convenient for gambling or card-playing or writing New York Times stories. Odds are used to express essential information about health benefits and risks. The odds ratio, for example, is an important way of communicating information about the relative benefits of one health intervention over another. Another way of communicating such information is through relative risk. In many common situations, these numbers are quite different, and the difference matters.
A highly simplified example illustrates this: Suppose that 18 out of 20 patients (90 percent probability, odds of 9:1) in an experiment lost weight while using diet A, while 16 out of 20 (80 percent, odds of 4:1) lost weight using diet B. The relative risk of losing weight by choosing diet A over diet B is 1.125, while the odds ratio is about 2.25.
The reasons a medical article might choose one method of reporting over the other are complex, but the message here is that sorting that out starts by being clear about the difference between probability and odds. As for your own writing, when in doubt, use probability rather than odds. Both concepts are often difficult for readers to grasp, but odds are usually harder.
Neither the relative risk nor the odds ratio is a sound measure of the strength of association reflected by two outcome rates because both the relative risk and odds ratio tend to be affected by the frequency of an outcome. I explained this in (1) a guest editorial in the Spring 2006 issue of Chance titled “Can We Actually Measure Health Disparities?” I explained the point most recently in (2) “Race and Mortality Revisited,” Society (July/Aug. 2014) and (3) a November 17, 2014 amicus curiae brief in Texas Department of Housing and Community Affairs v. The Inclusive Communities Project, Inc., Supreme Court No. 13-1371. Items (2) and (3) also explain that the soundest method for quantifying the strength of an association reflected by two outcome rates is the probit.
The relative risk, moreover, is an illogical measure as well as an unsound one, for reasons explained in item (2) at 339. See also references in note 12 at page 18 of item (3).
My December 2012 Amstat News Statistician’s View column titled “Misunderstanding of Statistics Leads to Misguided Law Enforcement Policies” discusses some remarkable anomalies in federal law enforcement arising from the failure of the federal government to recognize that reducing the frequency of an outcome tends to increase, not reduce, relative differences between rates of experiencing the outcome (while reducing relative differences between rates of experiencing the opposite outcome).
While the odds ratio is not a sound measure of association, it is not an illogical one. And it is closer to a sound measure than either of the two relative differences. As shown in Tables 3 and 4 of the Subgroup Effects subpage of the Scanlan’s Rule page of jpscanlan.com, for the critical task of applying an observed risk reduction in a clinical trial to estimate the absolute risk reduction (and corresponding number needed to treat) for groups with baseline rates different from that in the trial, the assumption of a constant odds ratio (Method 4) always yields an estimate closer to the probit estimate (Method 1) than an assumption of a constant relative risk as to either the favorable outcome or the adverse outcome. Depending on the actual shapes of the underlying distributions the odds ratios could provide a sounder result than the probit.
Caveat: On December 23, 2014, Yale Law Professor Ian Ayres filed an amicus brief in the above-referenced case that is highly critical of my brief. But, I think you will see that, whether or not the Ayres brief actually responded to anything in my brief, it (the Ayres brief) did not say anything pertinent to the points made above.
Each of the above references can be found in a second with a Google search.
Thanks to Ron for his observation. Dr. Scanlan’s remarks are based on the premise that a “sound” measures needs to be independent of the overall rate, which might be disputed. Also the probit is not an easy measure to explain to a non-statistician, even harder than the log odds (which is hard enough).
Nonlinear measures like the odds ratio do not have an obvious causal interpretation when applied to individuals. From a Neyman/Rubin causal model perspective, a simple and appropriate measure is simply the difference in proportions, which is the population average of the causal effect for an individual, the difference in the outcome (1 or 0) under the two conditions (e.g. treatments). One might well want to model data on the log-odds scale (as in logistic regression), but the predictions from the model can be the basis for a causal inference for individuals on the raw scale, that is the difference in estimated proportions, which is both appropriate and easy to understand.
The web site of a local establishment offered payoffs for football picks as follows: Pick 3 winners out of 3 picks yields a 6.5 for 1 payoff; pick 4 winners out of 4 picks yields an 11 for 1 payoff; pick 5 winners out of 5 picks yields a 20 for 1 payoff. Had that web site displayed it as “odds,” it would have been 5.5:1, 10:1 and 19:1 for the three scenarios. Said another way, the 6.5 for 1 payout may appear larger to those who confuse payout with odds. If you were to guess on the winners, the probability of picking 3 out of 3 would be 1/8 or 12.5%, 4 of 4 would be 1/16th or 6.25% and 5 of 5 would be 1/32 or 3.125%.
Thanks a lot. My confusion goes away.
For what it’s worth, while the article explains nicely the mathematical difference between the two concepts, I’m still at a loss as to whether I would ask my doctor for the probability of succcess or for the odds of success? Which one gives me a more useful way to choose?
A motivating discussion is definitely worth comment.
I do believe that you should write more on this subject
matter, it may not be a taboo subject but generally people don’t discuss such topics.
To the next! Kind regards!!