To err is human
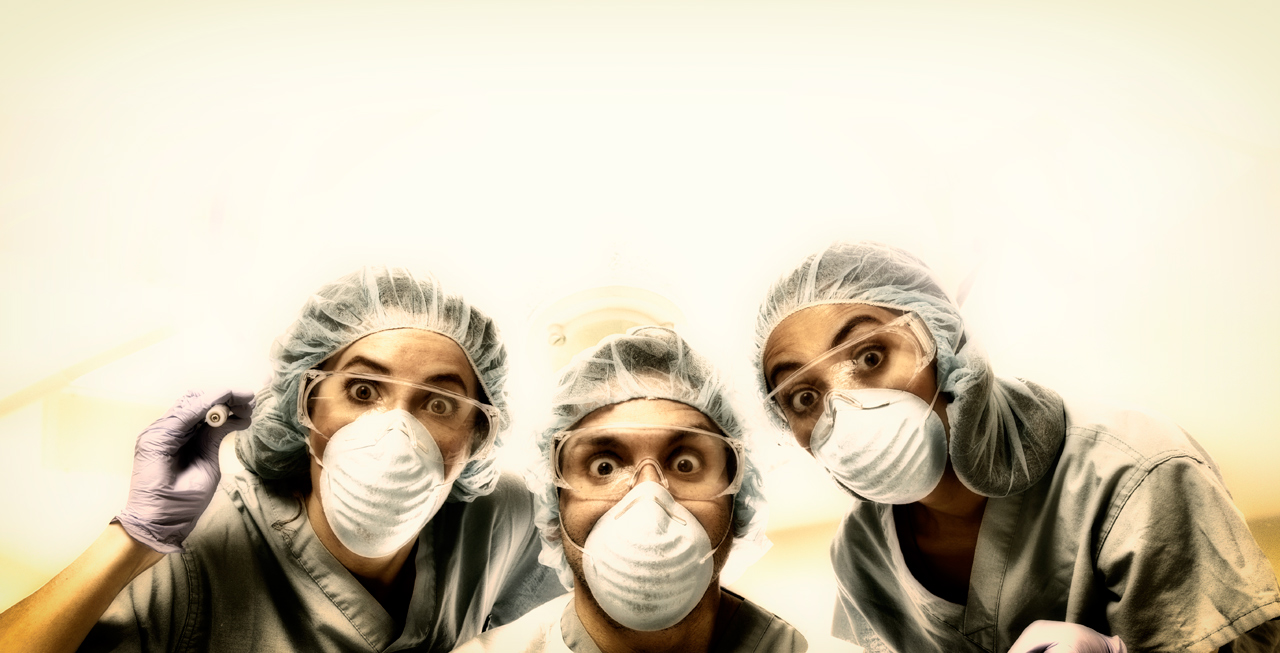
One of the oddest study results in recent years, one which you (probably) never heard about until now, must be the randomized control trial on massage therapy in which the participants—all adults—grew by almost two-and-a-half inches over eight weeks.
Odder still: no one—not the authors of the study, not the participants, not the editors of or the reviewers for the World Journal of Acupuncture-Moxibustion—noticed.
Perhaps it was because the question the study had been designed to answer—could massage induce weight loss—was, by itself, so startling. Those randomized to receive massage therapy lost ten pounds—ten percent of their baseline weight at the start of the study—compared to the control group, which didn’t receive any treatment.
As the mean weight of the study participants at the start of the study was about 75 kilos (165.3lbs), this meant that those being massaged were losing about two pounds a week.
“Really? 10 percent of your body weight at eight weeks? That seems like a lot from anything, even the best pharmaceuticals we have available,” said David Allison, distinguished professor in the Department of Biostatistics at the University of Alabama. “You’re telling me with a massage you can get people who aren’t very obese to begin with to lose that much weight?”
A sense of implausibility led Allison and his colleagues to pull apart the data and see how it added up. They “plugged and chugged” using geometrical means to analyze the study participants’ baseline, follow up weights and BMIs (Body Mass Index). Shazam! For the weight loss to occur, the control group needed to grow in height; and as they most certainly didn’t gain two plus inches in stature over eight weeks, they couldn’t have lost ten percent of their body weight.
The point of the exercise for Allison and his colleagues at Alabama was not so much to disprove a misleading finding as to see whether a significant error could be corrected.
They wrote to the study authors. The authors didn’t respond. They waited a bit and then wrote to the Journal editors. After some pressing, the editors contacted the study authors, and the authors eventually responded to Allison, admitting their error and correcting the data.
“But what was interesting to me,” said Allison, “is that they never explained how the error occurred and the editor never did anything about that. It’s one thing when you say there was a typographical error in one number in a table and that one number or the decimal point was moved. But these were a whole set of numbers; and you have to just sit there wondering—‘Well, aren’t you going to tell us how that mistake occurred?’”
The magical massage study was one of 25 examples where, over the course of 18 months, Allison and his colleagues attempted to correct “substantial or invalidating” errors they found in published research papers in obesity, nutrition, and energetics. As they compile a weekly newsletter of the latest research and articles of note, it wasn’t as much a case of them actively looking for errors as not being able to avoid them. We “genuinely wanted to help and clear up mistakes in the literature,” said Allison, “but we submitted our letters and we were just struck by the enormous variety of different things happening, of how confused the system seemed to be about how to deal with noting these large errors. We said, ‘Let’s keep it up for a while, learn a little bit, get some of these things fixed,’ and then eventually we just said, ‘You know what? We have to stop now. It’s sort of been interesting, but we just can’t keep doing this forever.’”
As they conclude in a recent article for Nature, the idea that science is self-correcting confronts a reality where actually getting a correction published—corrections that change the outcome of the study—is a challenge.
In one case, it took Allison and his colleagues just two weeks to analyze the raw data for a suspicious published study result, find a substantial error, and contact the editors of a journal. Eleven months later, the journal agreed to accept their letter and publish a retraction. Both are still awaiting publication. In another case, the editors of one unnamed journal suggested that Allison could simply post a comment online—or pay $1,716 to publish a letter in the journal explaining the error. Even in cases where the authors of a study were willing to swallow their academic pride and do the right thing by asking for their paper to be retracted, one publisher charges $10,000 to publish a retraction.
Of course, one might say that such responses are just insult being added to injury: why are all these broken papers being submitted for publication to begin with? In the majority of cases that Allison and his colleagues looked at there was either insufficient statistical input into the design of the study or erroneous statistical analysis and reporting of the data after the study had been done.
“We can’t say in the errors we’ve detected how many were intentional and how many were unintentional. We just kind of took at face value that most of them were unintentional and truly mistakes,” said Allison. “But I think as much as we would like it not to be so, we have to recognize that there are many pressures in the field of science. The interest in self-promotion, in fame, and in getting one’s next grant funded, or whatever, that lead investigators to want to make the most of their findings—to make them seem as impactful as possible.”
The answers to many of these problems are not insuperable: Register statistical plans for research before you do the research and describe how you did your analysis. Both of these principles are embodied in the National Institute of Health’s ClinicalTrials.gov and the CONSORT guidelines for reporting trials. But adherence or implementation is just not what it should be and, as a consequence, the incentives for researchers to put the nuts and bolts of their research up for public scrutiny are lacking. “We need to get to a more uniform playing field,” said Allison, “and get everybody to do it.”
More controversial is the need for researchers to publish or release their raw data. Only one of the examples Allison and his colleagues analyzed did so: “We got their raw data, we got IRB (Institutional Review Board) approval to use it, we quickly did analysis, and we were able to show that their conclusion was completely incorrect.”
“The temptation is to wag ones finger or chuckle at the mistake the investigators made,” he said. “But on the other hand, I praise them for putting their raw data out. By putting their raw data out there they allowed us to find the error and then they have since acknowledged it and that paper’s going to be retracted. I think that’s a great example of why we need as much raw data out there as possible.”
There are many good reasons for researchers to be reluctant to release their raw data. As Allison notes, you may not get permission from your Institutional Review Board, you may get scooped on findings that you yourself would like to make, and you may run afoul of those who want to discredit you by using your raw data to turn small innocent errors into evidence of malfeasance. “I think these are all legitimate concerns,” said Allison. “But we can’t make the perfect the enemy of the good. We have to move ever more to a greater and greater proportion of our raw data being publicly available.”
thats great, thanks
greeting from Afu University
http://goo.gl/tfeKBm